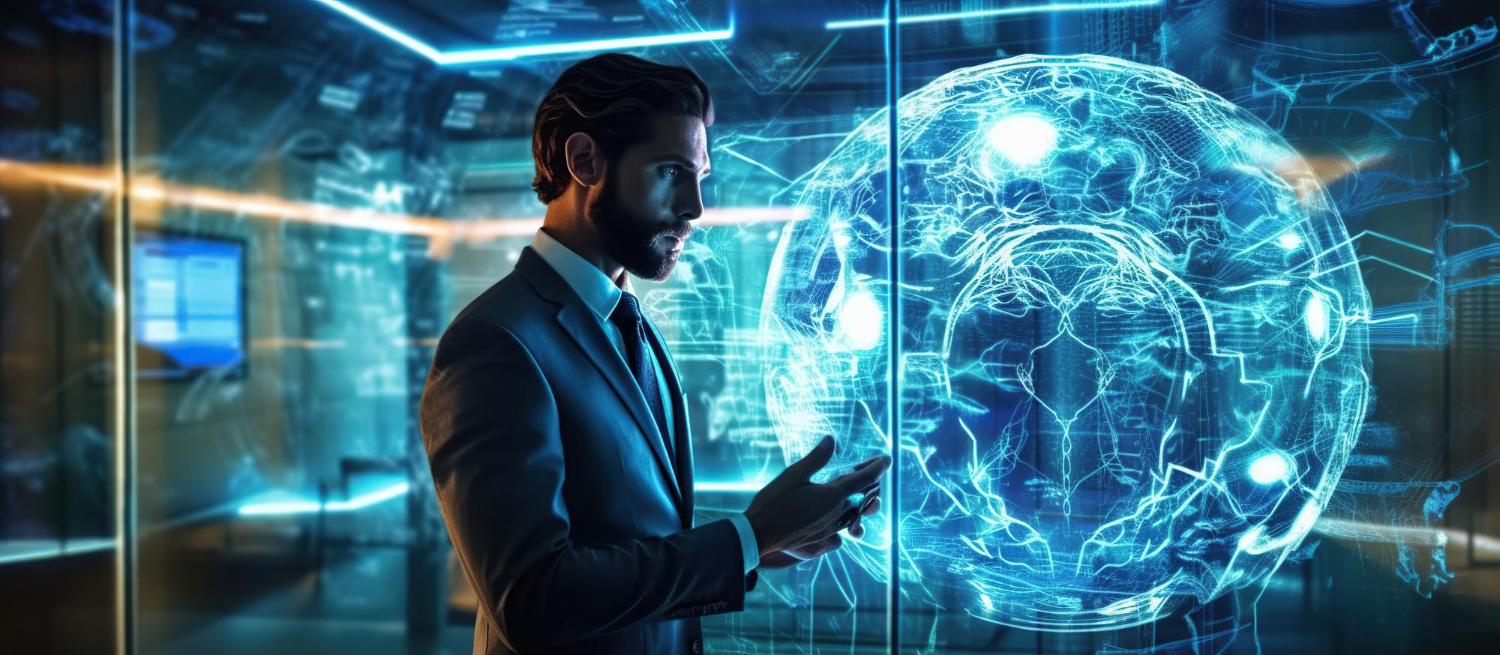
At TAZI, we are proud to share the success story of our journey with a $18B Wealth Management Company (refered as ‘bank’). The bank has achieved remarkable results in controlling customer retention and creating immense value. One of the bank’s champions even said, “Even if we save one customer per month, TAZI has paid for itself,” which made me think, “Damn it, we are underpriced!”
Our collaboration with the bank began with a strong-willed woman who firmly told her IT team, “You have been promising me a customer churn prediction solution for years now and haven’t delivered anything I can trust. I’m going to work with TAZI!” This determination secured the deal for us, and we immediately started working closely with their IT team, which became a valued partner in our solution.
This was our first venture into customer churn prediction for wealth management, and it has since expanded to various industries, including insurance, retail, banking, and telecom. Initially, we required a significant amount of training data, but with our solution’s use case library and the introduction of GenAI, we now achieve excellent results with minimal data. GenAI has significantly reduced training data requirements, but that’s a topic for another blog.
Kickoff: Defining Churn, Collecting and ETL Data, and Developing the Initial AI Model
In any financial services organization, data is sacred. To respect this, we installed TAZI on the bank’s servers. We formed a project team, planned deliverables, and started the process. We agreed on an initial data dictionary and prioritized collecting essential data for predicting churn, such as total assets transacted, number of transactions, commission rate, and total commissions paid. Additionally, we considered variables indicating past actions on churn, like outbound calls to customers and changes in the commission structure.
For our initial models, we defined churn as follows: if a customer closed their account within the next three months, they were labeled as CHURN; otherwise, REMAIN. We settled on a three-month prediction horizon, as one month was too short to take action and six months was too far ahead.
We processed the initial datasets through ETL, checked past data, and used this churn definition. But…
Iteration: Redefining Customer Churn with Many Shades
We had to decide whether to define retention at the account or customer level. We chose the account level, but the bank realized that defining churn based solely on account cancellations wasn’t enough. Some customers hadn’t canceled their accounts but had minimal activity, essentially indicating churn. We determined thresholds for small account balances and inactivity periods to trigger the churn label, checking the churn percentage distribution to make informed choices. Churn, it turned out, had many shades, including commission amounts.
Iteration: Checking and Improving Data Quality
There was a continuous process of data quality assessment and improvement. TAZI’s Data Profiler module assessed the relevance of each data column for predicting churn, providing statistics such as empty values, min, max, avg, and kurtosis (a customer favorite). These insights helped the bank’s marketing managers and business analysts understand churn behavior and data quality better.
Iteration: Handling Transactional Data and Temporal Changes
Some variables, like account balances and customer interactions, changed over time. Training data needed to reflect past situations, which slowed preparation. The bank had to “go back in time” to recompute variables, costing us a couple of months. Surprisingly, executives appreciated the learning experience, emphasizing the value of effort and learning over immediate results.
Iteration: Model Creation, Performance Check, Explanations, and Feature Engineering
Creating AI models on TAZI is straightforward. To evaluate performance, we used both machine learning metrics and business KPIs. We considered the value of a client based on commissions paid. The metrics screen showed the impact of actions on top predicted churners, guiding intervention strategies.
TAZI’s AI explanations highlighted the variables used by the model, fostering discussions between business and data teams. We adjusted variables based on relevance and transformed data types as needed. Automated feature engineering recommended useful transformations, like using the log of account balances, which business users appreciated.
To understand model behavior under different scenarios, we designed customizable dashboards for the bank. These dashboards allowed dynamic, real-time analysis, essential for immediate updates as models processed new data.
Going to Production
After months of testing and iterations, the churn prediction and prevention model was deemed production-ready. TAZI’s dashboards and APIs facilitated a smooth transition with minimal IT involvement. Today, we spend only a few hours per month on this model, ensuring its efficiency.
Handling Employee Churn
When the bank’s data scientist left, we supported the client until a new one was hired, ensuring no interruption in the model’s operation. Everything was already on the platform, making the transition seamless.
Value Created
The churn model, now operational and trusted, has reduced churn by up to 15% at different times. For example, if you have 10K customers, each valued at $10K/year, reducing churn from 10% to 8.5% saves $1.5M/year or $125K/month.
Additionally, the bank’s data is now cleaner and more accurate. The churn prediction models and their explanations improved data quality, helping business users identify and correct data processing errors.
The team has also learned valuable lessons. As the bank introduces new apps, they know how to store and process data for predictive AI models. Mistakes due to data quality or business changes are caught early, thanks to the collaborative efforts between business and data teams.
What’s Next for the Bank
The bank is ready for its next set of AI models, focusing on new data sources. Listening to clients more closely with Generative AI is another path forward, helping to increase customer happiness and reduce churn.